How likely am I to have COVID-19 complications? Machine learning could help predict the answer.
A team of Mines professors are developing computational tools to predict COVID-19 infections at individual, population levels
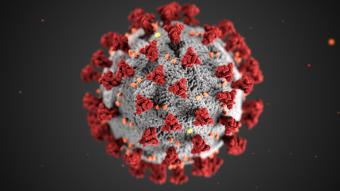
This illustration, created by the Centers for Disease Control and Prevention, shows the ultrastructural morphology exhibited by coronaviruses like SARS-CoV-2.
Colorado School of Mines professors are leading an effort to harness the power of machine learning in the fight against COVID-19 and the novel coronavirus that causes the dangerous illness.
Over the course of three months, more than 23,000 academic papers were published with findings related to SARS-CoV-2, the virus that causes the COVID-19 illness, said Hua Wang, associate professor of computer science. It is estimated that the number of published COVID-19 literature is doubling every 20 days — among the biggest explosions of scientific literature ever.
“No one can read all of them,” Wang said.
Enter machine learning: Wang and Judith Klein-Seetharaman, associate professor of chemistry and director of bioscience and bioengineering at Mines, are working with a team of health care professionals and technology partners to develop computational tools that can synthesize all of those findings as well as on-the-ground medical data to provide individuals and clinicians with the information they need to make decisions related to COVID-19.
“It is becoming clear that many factors are at play in who develops complications and ultimately dies from the infection, including molecular, physiological, lifestyle, behavioral, demographic and socioeconomic ones. In particular, comorbidities such as diabetes and high blood pressure are known risk factors for COVID-19 complications and death, but are likely only the tip of the iceberg. Molecular data indicates that as many as 100 comorbidities exist,” Klein-Seetharaman said. “Integrating large numbers of risk factors through machine learning will allow us to build statistical models that take all of the evidence into account – and hopefully predict COVID-19 infections at the individual and population levels.”
The ultimate goal, Klein-Seetharaman said, is to create an app that individuals (and, in the future, clinicians) could use to determine if they are likely infected with coronavirus and, if so, their risk of developing serious complications based on genetics and other clinical factors.
“We’re hoping to disseminate it to lots of people – individuals, as well as hospitals in Phase 2,” Klein-Seetharaman said. “Our models will be built on existing large data sets. The individual prediction will be based on the data the individual enters. What does it mean for you? It will be a completely personalized output.”
Local health care technology firm Ingenious will build out the app. Medecipher, a Denver-based health IT company that provides clinical operations support for health care providers, has offered up its servers to store the data and model. Both are tenants of Catalyst HTI, the Denver health care innovation hub where Mines also has an office and Klein-Seetharaman leads an initiative for artificial intelligence in bio and health. “It’s through the Mines@Catalyst office that we have these connections,” Klein-Seetharaman said.
At Mines, Wang is an expert in what’s called the “missing data imputation problem,” one of the major challenges in developing computational tools like this from diverse data sources.
“The true challenge is the data is collected from different agencies and by different types of instruments. All this data is collected in different ways. They are not formatted and aligned well,” Wang said. “Machine learning is great for integration and knowledge discovery. How to utilize the multiple sources of information effectively to make predictions for the development, progression and final result of a SARS-CoV2 infection – it’s a challenging question. It’s not easy to handle with traditional mathematical and statistical methods. That's why machine learning could help.”
Funding for the one-year project comes from the National Science Foundation’s Rapid Response Research (RAPID) program. It is one of two coronavirus-related NSF RAPID grants Mines researchers have been awarded in recent weeks.
John McCray, professor of civil and environmental engineering, is leading the other COVID-related project, which will study the impact of Colorado’s Stay-at-Home orders – and ubiquitous working from home, online education and online shopping and delivery – on urban stream quality in the Denver metro area.
“The COVID-19 pandemic could potentially accelerate future sustainable living practices into typical living scenarios,” McCray wrote in his proposal. “Research is underway looking at air pollution but little has been done to understand the impacts on water quality. Cleaner, fishable and swimmable urban rivers would be another justification for sustainable living that includes working from home and much less driving. The information will also be useful to urban planners regarding types of green infrastructure for cleaning urban water, and to public health officials and legislators for urban water quality management.”