Mines faculty teaching robots how to think, share information
Xiaoli Zhang, associate professor of mechanical engineering, is using machine learning to improve manufacturing processes
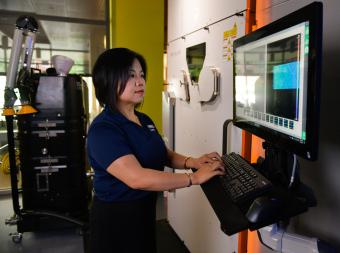
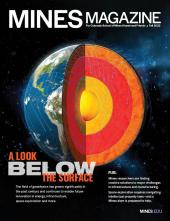
Many manufacturing processes today rely on 3D-printed methods and machine learning to produce high-quality products at a faster rate. This requires machines that can evolve and improve over time through learned experience. But getting machines to share knowledge is easier said than done.
We asked Xiaoli Zhang, associate professor of mechanical engineering, to tell us more about how machines can learn and share knowledge to improve manufacturing processes. This is what she shared.
Training machines is like teaching human babies.
When 3D printing a part or product, most of the quality control, such as determining the hardness of the printed material, is completed after the part has been completely manufactured. But Zhang is developing process-structure-property (PSP) machine learning-based models to identify defects in real time to avoid failures and save time and money.
To get a machine to successfully adopt a PSP model, researchers feed it data, and the machine-learning techniques will explore that data to build PSP correlations on its own. Scientists approach this in a similar way that humans teach babies to recognize the differences between images of cats and dogs.
When babies are given images of cats and images of dogs, they can quickly learn to distinguish them—something machine-learning models need to learn as well.
“We give the data to the machine learning algorithm, and we provide it with a ground truth—we tell the computer the correct answer at the beginning of the training,” Zhang said. “Just like a baby can figure out the difference between a cat and a dog quickly, the computer machine-learning algorithm will also learn how to distinguish them given the ground truth. This way, once a machine-learning algorithm is trained, it will be able to conduct defect classification or quantification tasks, even the given data that are different from the original training data, just like a baby can differentiate new cat images from dog images.”
Once a machine understands these ground truths and the parameters needed to successfully print a part or produce a product in a specific way, it can make adjustments in real time and avoid mistakes during the characterization process, eliminating the need to complete post-characterization tests to determine the quality of the product.
Getting machines to learn from each other requires a shared brain.
The next step in improving additive manufacturing processes is getting the machines to communicate and learn from each other without humans having to upload data to individual machines. Zhang has a solution that was also inspired by humanity: a system that functions similarly to how humans share knowledge via the internet.
“In terms of the machine knowledge transfer, once we have a model in one printing machine, we want to transfer that to another one,” Zhang said. “Think about how we develop our human world—we learn from each other and share through the internet. Machines can also have their internet with a cloud-based system. Each machine can post its data and knowledge, run it through the cloud and then it can be assigned to every machine in communication with the cloud. Then they can share and learn from each other.”
When a machine uploads a PSP model to the cloud, other machines on that same system can access that model, learn the specific parameters required to produce a part and successfully print the product with the desired characteristics. Humans wouldn’t need to manually upload data or teach individual machines how to make the appropriate correlations from scratch. This interconnectivity and shared knowledge can help manufacturers scale up the number of products and capability for mass production, cut labor costs and decrease production time.
“I imagine we will have less and less labor work to do the monitoring and post-characterization, but that doesn’t mean it’s a negative,” Zhang said. “We can produce products faster, and then the overall workforce development may grow.”